3 Ways to Leverage Generative AI in Pharmacovigilance
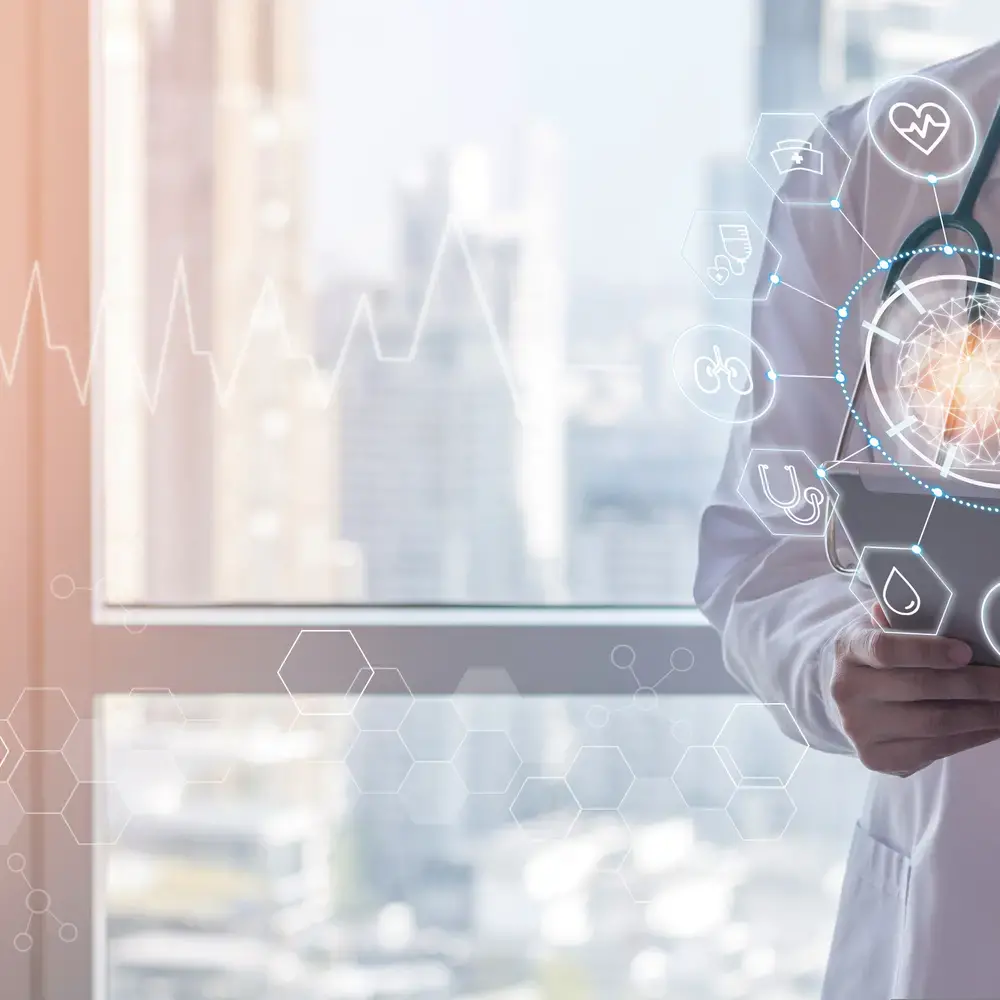
Despite the rigorous drug safety and efficacy standards in trials, study medicines can have unintended side effects on patients throughout the clinical trial journey and even in the post-market setting. To ensure patient safety and mitigate risk, global health regulatory authorities such as the US Food and Drug Administration (FDA) and the European Medicines Agency (EMA) require that any adverse events are identified and reported. To meet the FDA’s requirements in the US and the EMA’s requirements in the EU, clinical and regulatory teams must initially report fatal adverse reactions within seven calendar days and serious yet non-life-threatening events within 15 calendar days.
Pharmacovigilance plays a key role in ensuring medicinal product safety and improving patient outcomes. According to the World Health Organization, pharmacovigilance (PV) involves the science and activities relating to the detection, assessment, understanding, and prevention of adverse effects or any medicine/vaccine-related problem. It’s no question that the manual process of synthesizing and reporting on medical information can be a complex administrative burden. In fact, it’s estimated that only 1–10% of adverse effects are actually reported.
PV will always be essential for drug approval, continuance, and safe promotions. To meet health authority guidelines and combat rising costs, PV and safety teams will need to innovate with cutting-edge technology. Let’s examine three significant ways the use of artificial intelligence, GenAI, and machine translation in PV processes and documentation can mitigate risk and cost for pharma organizations.
Safety Intake and Case Triage
As an essential first step in PV and for submitting PV system master files (PSMF) down the line, safety intake involves collecting large volumes of data about adverse drug reactions from various sources. After initial medical information data collection, teams focused on PV and safety triage the adverse event cases. They categorize cases by receipt date, severity, causality, and the corresponding expectedness assessment. Within this overall process, teams are also tasked with the time burdens of verifying, validating, and entering data within secure databases.
Historically managed manually, the drug safety and PV data process can be prone to human error and inconsistencies in resulting reports. By leveraging GenAI and training it on historic datasets, teams can automatically synthesize research data and generate accurate, summarized adverse event reports for their regulatory content submissions to health authorities. AI-powered machine learning models can even convert unstructured data to structured data. This results in accurate and unbiased data that improves operational efficiencies and maintains good pharmacovigilance practices (GVP) compliance. With an increasing influx of adverse event data, teams can utilize artificial intelligence to traffic adverse event safety notifications and distribute alerts in real time. Overall, this use case helps to improve the speed and accuracy of PV and safety reporting.
Automated Chatbot Localization
Once a medication enters the global market, patients and healthcare professionals alike may have queries about the product’s medical and safety information. In the past year, emerging research found that chatbots were able to resolve 75% of queries, introducing potential for the PV and safety landscape.
By utilizing a GenAI-powered natural language processing chatbot trained on accurate contact responses, patients and HCPs can receive automatic, real-time responses to their drug safety questions when they need it the most. By using machine translation for localization in consumers’ native languages, PV and safety teams can increase readability across global markets while ensuring messaging remains compliant and culturally appropriate.
Literature and Social Media Monitoring
To build accurate reports on a medicinal product’s safety and efficacy, it is essential to comb through published and unpublished literature discussing adverse events. By leveraging AI-powered workflows, PV and safety teams can crawl through keywords across global publications in any language and report back to a centralized platform for human review.
In recent years, the “literature” within PV literature monitoring has expanded to include user-generated social media content. In some cases, patients and HCPs alike may feel more empowered to anonymously share their medication experiences online than in an in-person clinical setting. By training a GenAI model, WHO-UMC researchers found they could effectively automate the identification of adverse drug reactions from social media posts, specifically monitor for safety signals, and complement traditional spontaneous reporting methods to offer additional insights. There’s a promising continued future use case for AI in social media listening for synthesizing adverse event data from historically underrepresented groups and rare disease communities.
Conclusion
The use of generative AI in pharmacovigilance and safety has transformative potential for life sciences organizations. Increased speed and reduced errors can be lifesaving in the context of adverse event and medical safety data reporting. In the future, some researchers posit that intelligent GenAI models may be able to predict and forecast adverse events before they even happen, which could be a game changer in the PV and safety landscape.
Backed by 21 CFR Part 11-compliant technology, TransPerfect Life Sciences leverages pharmacovigilance automation to improve the speed and accuracy of PV and safety reporting. If you’re interested in a 40% reduction in cycle times and 50% faster redactions, reach out to our team to learn more.