Implications of AI in Healthcare and Life Sciences: Ethical Considerations in the Age of AI
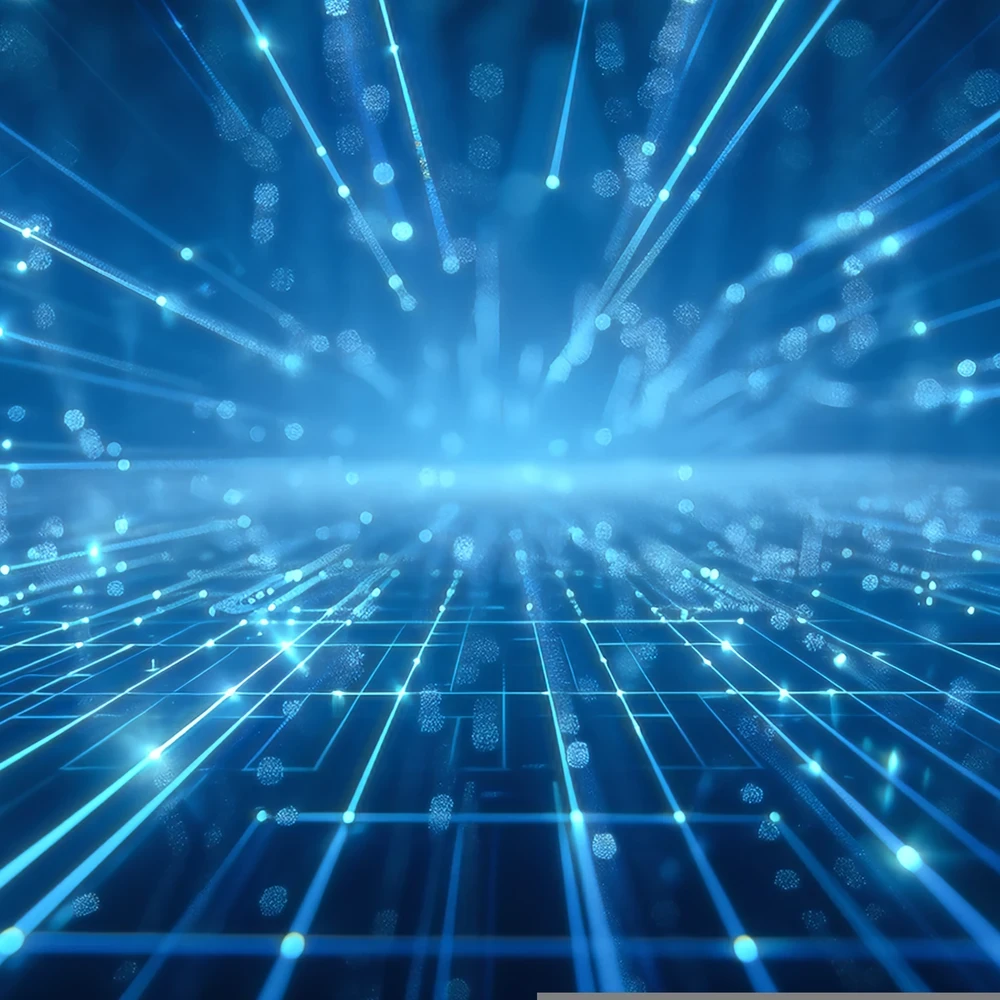
Artificial intelligence (AI) models have already shown transformative potential in the life sciences industry, including automating administrative clinical research tasks, analyzing patient data to predict future health risks, synthesizing medical information, and more.
Healthcare authorities are staying on the pulse of AI’s continued potential, with the US Food and Drug Administration (FDA) exploring benefits for streamlining workflows and reducing time-to-market and the European Medicines Agency (EMA) publishing its official five-year workplan to embrace AI in regulatory productivity.
To better understand the nuances of this complex conversation, let’s examine three of the most significant ethical considerations for AI in healthcare. We’ll also discuss strategies for mitigating any potential risks.
Data Privacy and Security
To support clinical and regulatory teams’ research goals, AI-powered workflows need to analyze volumes of sensitive and confidential health data. Even with the ease and speed of automation, leading life sciences organizations must keep data privacy and security a top priority in all processes.
As a first step in the clinical trial process, especially during the informed consent phase, researchers should disclose to patients the potential risks involved with using AI to collect their data in the study. To ensure data privacy compliance once data is collected, it’s important to implement strict cybersecurity measures such as encryption, access controls, and regular security audits. These measures can help protect AI systems from any breaches. Human oversight, including data supervision, quality assurance, and reviews for HIPAA compliance, should always be considered to maintain ethics while using AI in healthcare.
Bias and Fairness
“AI hallucinations” occur when generative AI models provide teams with fabricated data outputs that appear authentic. This phenomenon can happen when GenAI is trained on biased or inaccurate data points, since the system can’t verify the accuracy of the content it receives.
To further mitigate bias in the data collection and model training process, teams must prioritize diversity and inclusion in their clinical trials to collect various perspectives for the AI model to analyze. As an additional strategy to consider ethics and fairness while using AI, humans must be in the loop to critically analyze and cross-check any data outputs against expert publications. Clinical and regulatory teams can also perform ongoing audits to detect and monitor any bias vulnerabilities in the AI model’s design.
Transparency and Explainability
Clinical and regulatory professionals may be familiar with the concept of a “black box” in AI. Simply put, black box AI refers to machine learning models with internal decision-making operations that users can’t fully understand or see. With rapid conclusions, minimal computing power, and increased automation efficiencies, it can be advantageous to leverage black box models. In some cases, after being trained on EHR record training data, black box AI models surpassed human doctors in accurate predictions of rare disease diagnoses. However, due to the lack of explainability, it’s not entirely clear if bias factored into the model’s outputs, and patients could receive inadequate medical information from professionals who rely solely on the black box AI’s judgments.
While this concept is still under study, clinical and research teams can prioritize transparency and explainability when utilizing any black box AI models. In addition to using the aforementioned strategies to uphold data security and mitigate any bias in black box AI, teams can rely on simpler, interpretable AI models to explain the outputs of more complex systems.
Conclusion
In the highly regulated healthcare landscape, emerging technology must always be examined thoroughly to ensure the most ethical and responsible use cases. By prioritizing data security, mitigating bias, and upholding transparency when utilizing AI models, clinical and research teams can truly help improve global healthcare outcomes.
Concerned about the ethical considerations for leveraging AI in your clinical and regulatory processes? Reach out to our team today to learn more about our AI data solutions.