Leveraging Technology and Bridging Gaps for Enhanced Clinical Data Management
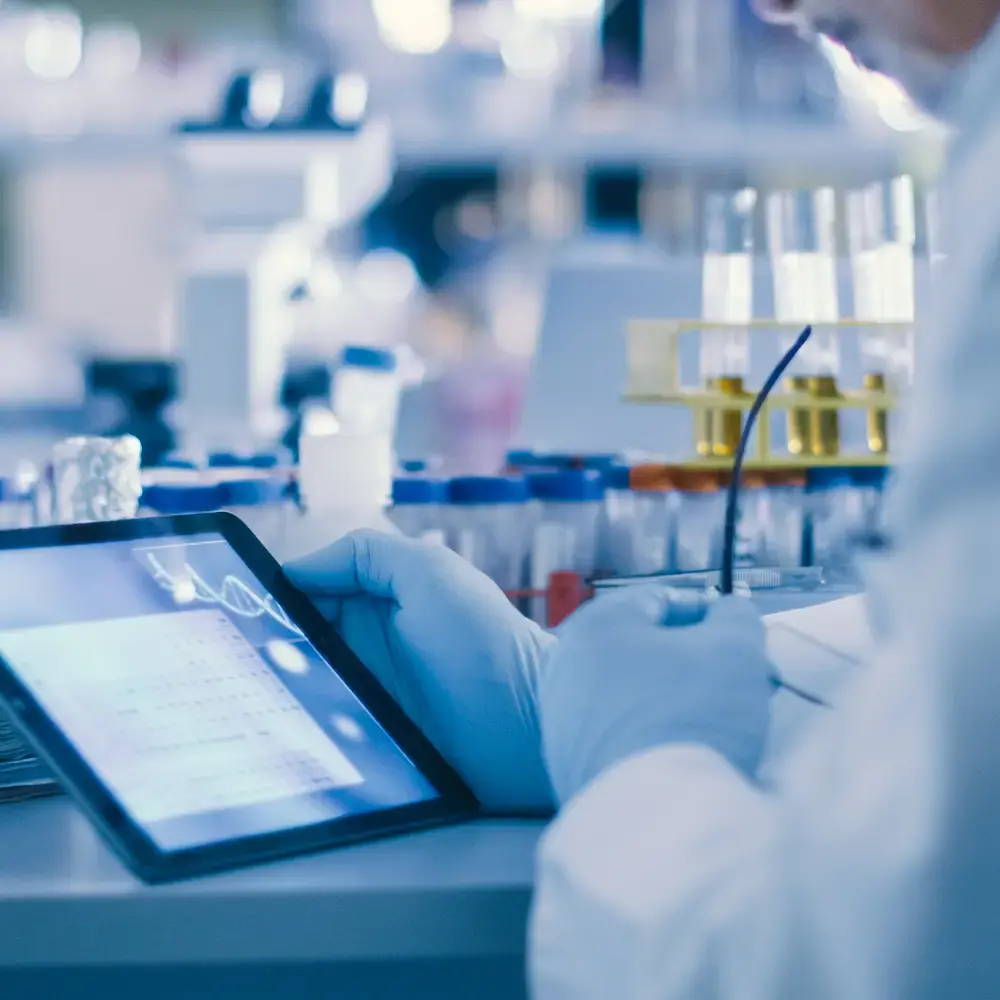
Introduction:
In the field of clinical research, a robust data management process is essential for driving success. Efficiently handling vast amounts of data, ensuring accuracy and consistency, and extracting meaningful insights are vital, particularly in the case of global clinical trials.
The volume of data collected throughout a clinical trial is dependent on several factors, such as the trial scope, the number of participants, and which endpoints are being evaluated. This includes structured data, such as patient demographics and lab results, as well as unstructured data, such as trial notes, imaging files, and patient-reported outcomes. In recent times, an increase in digital health technologies aims to keep up with the growing volumes of data collected, both in real-world and clinical applications.
It is, nonetheless, becoming continually complex to manage, store, analyze, and act on this data without an effective assessment of existing technology frameworks and management strategies. Research teams should assess their existing processes and identify potential gaps, which may be creating inefficiencies or incurring high costs/resources.
Current Industry Trends and Problems in Clinical Data Management Workflows:
As datasets continue to grow, so do the many challenges that can impact how efficiently the data itself is leveraged on a broader scale. Some of these complexities include:
Manual Data Entry and Verification:
Spending extensive hours inputting data from paper forms or disparate electronic sources is a time-consuming, error-prone process, often resulting in inconsistencies or inaccuracies. Manual verification of this data exacerbates the issue, causing delays and jeopardizing data quality.
Teams that rely on manual data may face complications when reconciling and cross-checking data across multiple sources, leading to discrepancies and requiring extensive data clean-up.
Disparate Data Sources and Inefficient Integration:
Clinical trials generate large datasets from various sources, such as electronic health records, patient-reported outcomes, lab results, and wearable devices. However, these data sources often operate separately, making it difficult to integrate and harmonize the data. An inefficient integration process can result in fragmented and siloed data, hindering the ability to derive comprehensive insights. Furthermore, teams may find themselves duplicating efforts, increasing time consumption, and potentially missing critical data points.
Inadequate Data Standardization and Governance:
Non-standardized data collection processes and inconsistent formats may hinder efforts for efficient data management. A lack of proper data governance frameworks and standardized protocols causes data quality, comparability, and interoperability to suffer. Ultimately, this makes it difficult to conduct meaningful cross-study analyses or leverage data for post-trial analysis and real-world evidence generation. Furthermore, difficulties that arise when aggregating and analyzing data across multiple sites or studies can impact research outcomes.
Limited Utilization of Automation and AI/ML Technologies:
Underutilizing automation and advanced technologies, such as artificial intelligence (AI) and machine learning (ML), is another roadblock to effective clinical data management. Manual and repetitive tasks, such as data clean-up and adverse event identification, are optimal candidates for automation using AI/ML algorithms, with the outcome of improved efficiency and reduced human errors. Without leveraging these algorithms, researchers could spend valuable time and resources manually reviewing adverse events, leading to potential delays in identifying and highlighting safety concerns.
Bridging the Gaps with Technology-Driven Solutions:
In clinical research, selecting one system over another may not be as straightforward as it seems. In many cases, a combined approach that outlines clearly defined roles for respective systems can maintain existing frameworks while bridging gaps where necessary. Acknowledging current limitations can guide a more effective evaluation of which systems should be implemented.
Once clinical teams have conducted a thorough analysis of existing systems, there are several technology-driven solutions available to streamline workflows, enhance efficiency, and optimize data management processes. Some examples include:
Component Content Management Systems (CCMS):
A CCMS is focused on content details, or “components,” in files designed to centralize document management. By centralizing all clinical content, such as investigator brochures, protocols, patient consent forms, and regulatory materials, a CCMS enables information storage and retrieval.
In cases of multilingual clinical materials, the system’s content reuse capabilities enable researchers to establish consistency across trial materials by tapping into and repurposing pre-approved content. This subtracts the need for further data compilation and duplicated creation efforts while reducing the time and costs associated with creating content from scratch.
Translation Management System (TMS):
A TMS leverages a centralized database known as a translation memory (TM), which stores existing content and translated segments, including clinical technical terminologies or specific languages. This enables translators to reuse pre-approved content and ensure consistency across multiple languages. This also eliminates the need for data compilation and duplicated creation efforts. As a result, the time and costs associated with creating content from scratch are significantly reduced.
By reducing turnaround times and optimizing the translation process, TMS tools enable researchers to focus on critical tasks and improve overall operational efficiency. Moreover, the automation and standardization provided by TMS eliminate the administrative burden associated with translating materials for different sites, resulting in cost savings and increased productivity.
Clinical Trial Management Systems (CTMS):
Clinical Trial Management Systems (CTMS) play an essential role in alleviating the burdens associated with clinical trial data management. A comprehensive CTMS platform offers a range of features designed to streamline workflows by centralizing trial information and offering real-time insights.
With a CTMS, research teams work out of a central system that integrates with other technologies, such as document management systems, electronic data capture (EDC), and reporting/analytics tools. These integrations create a holistic ecosystem that enables researchers to monitor multiple clinical activities throughout the trial lifecycle, eliminating the need for manual data entry and providing visibility throughout all stages of a clinical trial. The automation of data collection and tracking in a CTMS also reduces the administrative burden on trial personnel, allowing them to focus more on critical tasks and data analysis.
Moreover, CTMS platforms can track key performance indicators (KPIs) to provide insights into trial progress and measure performance. By generating comprehensive reports and analytics, a CTMS can empower trial teams with actionable data to make well-informed decisions and identify potential gaps in the process. Incorporating a data-driven approach through a central technology framework can ultimately improve process efficiencies, accelerate timelines, and contribute to the overall success of clinical trials.
Artificial Intelligence and Machine Learning Technologies:
AI and ML technologies have transformed the way clinical trial data is processed and analyzed. These tools can rapidly sort through large amounts of clinical data, identify patterns, and extract meaningful insights. For example, predictive analytics tools can optimize patient recruitment and retention efforts, identify potential safety risks, and empower more data-driven approaches to decision-making. AI-powered algorithms can also automate data clean-up, minimize human errors, and ensure data accuracy.
Furthermore, AI/ML tools can identify key data points in the discovery and development phases of clinical research, including relevant biomarkers; stratify patient populations; and assess potential treatment responses. By identifying specific patterns and correlations in large datasets, AI systems offer critical insights into disease mechanisms, treatment efficacy, and adverse events. These contributions accelerate the drug discovery and development processes, demonstrating how AI/ML frameworks improve research data and, consequently, patient outcomes through more efficient and effective clinical trial management and analysis
Conclusion:
Research teams seeking to overcome challenges, improve efficiency, reduce costs, and accelerate timelines should assess how these solutions may fit into existing frameworks and alleviate some of the pain points in their clinical operations. Automation, advanced technology, and centralized systems enable researchers to focus on critical tasks and drive better patient outcomes while keeping costs low and turnaround times on track.
By leveraging technology effectively, researchers can unlock the full potential of data, leading to more advancements in clinical research and improved healthcare outcomes.
Want to get started with optimizing your clinical operations and maximizing your research teams’ abilities? Reach out today to learn how TransPerfect Life Sciences can inform your data management strategy.