Leveraging Artificial Intelligence (AI) in Pharmacovigilance
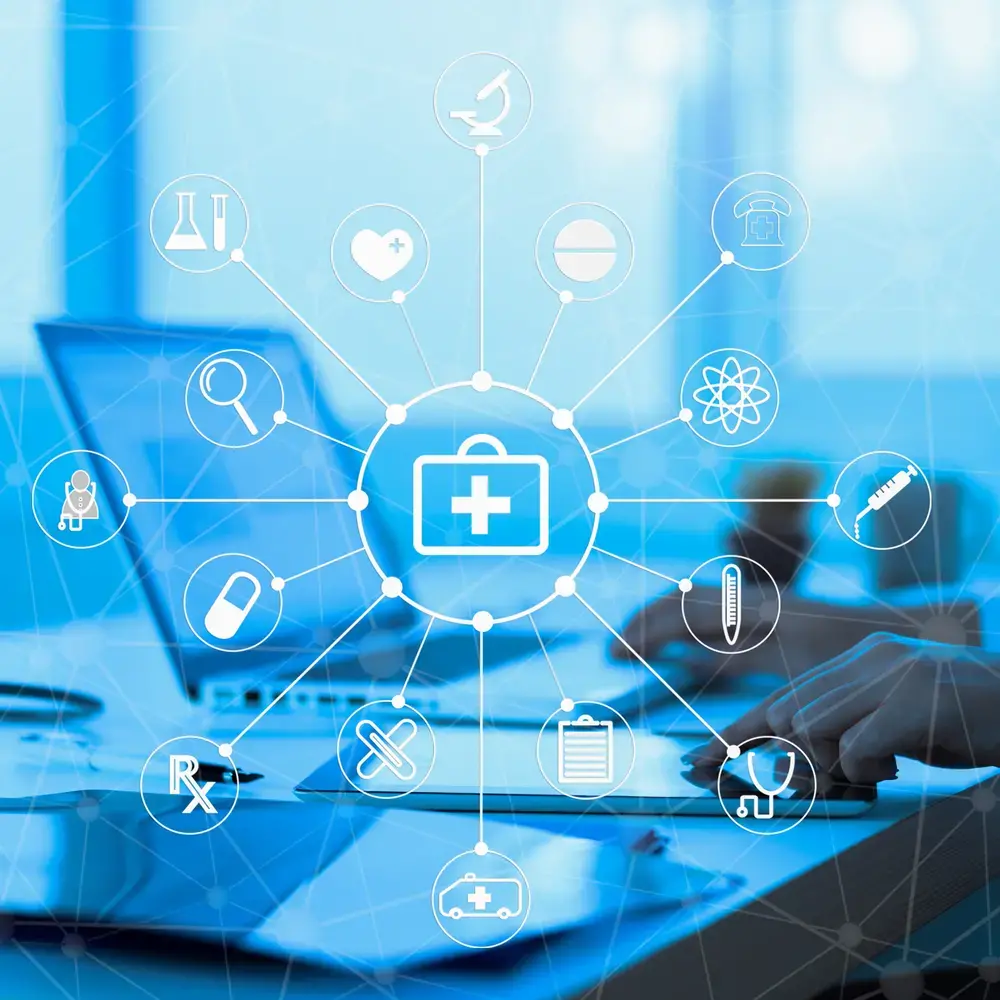
Expert-driven technologies are continually being implemented into the clinical process to eliminate manual burdens and ramp up efficiencies. AI-enabled automated systems help to remove the barriers that prevent high-quality data and compliance in reporting of clinical events.
Many mid- and large-scale pharma organizations are investing in AI-enabled automated pharmacovigilance (PV) processes, applying algorithms to data entry and triage, medical review phases, and reporting. In doing so, they aim to enhance data quality and accuracy, eliminate bias, reduce the manual burden on their teams, and maintain compliance with regulatory requirements. Providing real-time monitoring of high-quality data sets also has significant implications for patient safety and protection.
Organizations are adopting a proactive approach to risk management through AI applications throughout their PV life cycle, from case intake to reporting. The two main categories for leveraging AI in PV processes are the insertion of unstructured content and decision-making AI. Both are beneficial for their respective uses, reducing processing and reporting times. However, the discussion surrounding its use in PV remains sparse.
Opportunities for AI in PV
Case Intake: Ever-increasing volumes of unstructured data present a key challenge for PV teams when managing case intake. Processing inbound case safety reports (ICSRs) can take anywhere from a few minutes to several hours. Natural language processing streamlines data extraction, while AI, such as optical character recognition (OCR), converts unstructured content via Docx, XML, PDFs, etc., to prioritize cases based on severity and relevance.
Teams can then make manual adjustments or confirmations that are re-routed into the engine to increase accuracy and consistency in future use. This process reduces cycle times for case processing, enhances safety databases, and provides future-proofing and scalability in managing growing volumes of case data.
Local Literature Monitoring: Since regulatory bodies require routine literature searches to be completed in a certain timeframe, and many organizations struggle with local search efforts, implementing AI can expedite multilingual data extraction and reporting. This process automates source location and consolidation into one data set for seamless application of search strings. It also centralizes literature reviews and identifies required actions for easy translation management and content submission and delivery. Through automated sourcing of relevant literature and identification of keywords, PV teams can see what data has been extracted for review and reporting.
Case Processing: A key goal for these organizations is overhauling the cost of case processing, which translates into roughly 45–80% of spend and causes a significant resource drain due to high variability in narrative writing. By automating the event narrative, the AI rapidly generates a similar cadence as a human reviewer and places it into an assigned template, thereby maintaining consistency and standardizing the process. Audit tracking and version control provide complete visibility into all phases of the process. In turn, this facilitates streamlined reporting, alleviating strain on PV teams.
Predictive Signal Detection: While most organizations continue to leverage traditional methods through database mining and assessment of individual spontaneous adverse events reports, others have begun working with real-world evidence. Overall, the goal for organizations looking to implement more automation into the process is predictive signaling. AI grabs and analyzes data from multiple source points, including patient demographics and therapeutics, in real time. This provides PV teams with more resources to assess and validate potential signals. Risk management plans can then be created based on related data, edging closer to a future of predictive PV.
Combatting Potential Limitations of AI in PV
AI promises more efficient workflows and streamlined, higher-quality data sets to optimize the PV process, but there are equally certain question marks about the extent to which it can or should be used. Despite rapidly evolving technologies and robust machine learning, there are concerns about the quality of information, how accurately the engine can interpret it, and how to justify decisions made by the AI.
Key limitations of AI in the PV process include:
- Quality and accuracy
- Data privacy and security
- Availability of training data
- Data imbalance leading to potentially invalid conclusions
There are also concerns about the vast quantity and diversity of data sets required to adequately train AI, which may be arduous and resource-intensive to compile. In addition, much of the research obtained may be retrospective, creating training materials that are incongruent with current data.
With the gold standard of human expertise posing a key challenge for the further deployment of AI and ML in the PV process, guidance points toward tech-enabled human intelligence to combine respective efficiencies. By automating the manual, labor-intensive work, teams have more time to assess the accuracy and quality of reporting, creating an effective, balanced workflow that optimizes each phase of the PV process.
Conclusion
The practical use of AI in pharmacovigilance allows for substantial quantities of safety information to be collected in the post-market phase of drug development. The application of AI in PV is in its infancy, with discussion surrounding it yielding a number of concerns about its capabilities and limitations. As AI workflows are further implemented and the technology continues to evolve and advance, these processes can save PV teams resources, time, and money.
Contact us at lifesciences@transperfect.com to discuss how your PV and safety teams can benefit from AI.