How Life Sciences Companies Can Detect Adverse Events on Social Media
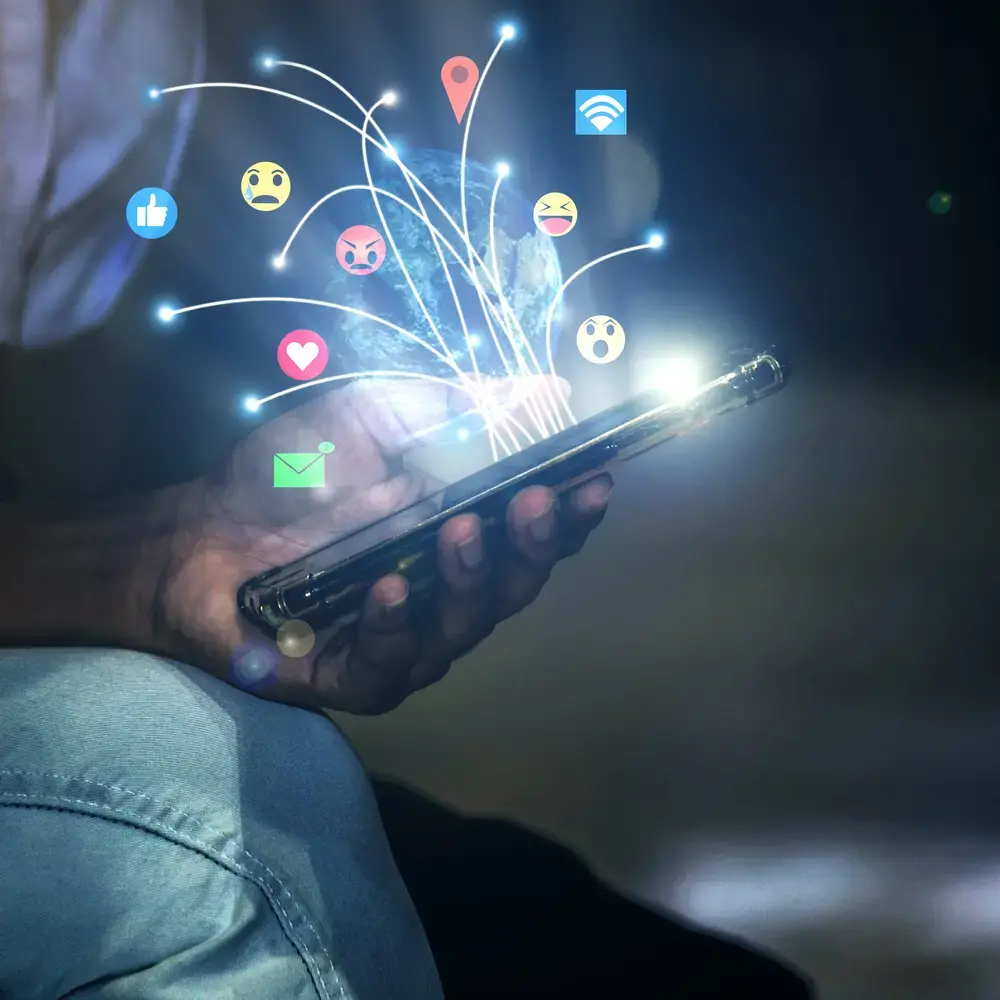
Pharmaceutical companies have historically relied on email correspondences, company websites, and physician hotlines as adverse event reporting channels. However, the incredibly high usage of social media means it is no longer a channel that can be ignored. Although safety departments haven’t utilized these channels before, social media has become a source for post-marketing safety information.
So what caused this change, and what can we expect moving forward?
Adapting to Social Media
The rapid expansion and immediacy of social media websites, such as Facebook and Twitter, provides broad coverage of health-related topics. Pharmaceutical companies have adopted more digital technologies and media in order to communicate with patients and create awareness about diseases, treatments, and support programs.
Social media tools allow for an easy exchange of content and encourage knowledge sharing.
In fact, recent surveys suggest 90% of people between the ages of 18 and 24 trust medical information shared on their social feeds. Additionally, 77% of individuals use a search engine as the primary tool for finding medical advice. Given their ease of use and accessibility, both the internet and social media have high potential for faster signal detection.
According to the guidelines on Good Pharmacovigilance Practices (GVP), social media can be a source of potential valid Individual Case Safety Reports (ICSRs). These reports allow patients and healthcare professionals to communicate adverse drug reactions, proving social media’s use as a source for new signal identification.
GVP Module VI states that marketing authorization holders should regularly screen the internet or digital mediums for potential reports of suspected adverse reactions.
As a result, social media monitoring will likely become a standard practice in the pharmacovigilance (PV) future.
Challenges of Change
Companies have the ability to transform social media platforms into strategic PV tools.
Two major advantages of using social media data over traditional AE reporting data include:
- Direct access to real-time data
- Potentially richer sources than reports filtered through HCPs
On the other hand, there are several challenges to consider:
High Volume of Data
Social media produces large amounts of raw data that can be difficult to analyze. The challenge is to quickly identify the relatively small number of adverse events from these large amounts of data.
Accuracy of Information
Social media users may not share medical data in the same way they would with their doctors or healthcare professionals. Many posts do not describe symptoms using proper medical terminology that would explicitly confirm certain conditions or diagnoses. Also, drugs may be referred to using creative idiomatic expressions or terms not found within existing medical lexicons.
Privacy/Data Protection
Safety data collected via social media often contains personal data related to the patient and the person writing the post. This can include information about the patient’s healthcare provider, family members, or the patient themselves. Using this type of data increases the risk of data breaches and cyber-attacks.
Filtering
Although there are statistical, semantic, and other methods to accelerate data processing, filtering the right data can be a gargantuan manual task.
False positives (content wrongly identified as an AE) and false negatives (content that should have been classified as an AE, but wasn’t) constitute a large portion of the results (often greater than 10%).
False positives may come up for many reasons. For example, a user review may mention a different drug. On the other hand, false negatives may occur because a user rates a drug highly while also mentioning adverse events.
How AI Can Help
Natural language processing and other artificial intelligence applications can help find adverse events and reduce manual revision of extracted records.
The latest research shows that state-of-the-art models and algorithms can improve accuracy and reduce noise and false negatives. Industry-grade language modeling requires a large amount of labeled training data, which can be hard to obtain at a reasonable cost.
Luckily, there are specialized tools, metrics, and processes that can help create high-quality labeled datasets relatively quickly and at the required level of accuracy. These include specialized micro-task management platforms with text annotation and quality assurance features, as well as blind annotation processes with reconciliation steps.
TransPerfect DataForce offers AI solutions for the life sciences industry. Contact us today to learn how we can help.